Information is something powerful in the age of the internet and the massive dissemination of content. However, more important than the information itself is the interpretation we offer it. These tons of articles, opinions and data that reach us must have an analytical filter, a skeptical critique, and for this we must resort to science to evaluate and make the best decisions and issue opinions, especially if the focus is on decisions and public policies that affect thousands of people.
Therefore, understanding where, when and, above all, how to interpret the information we receive becomes very important, from an individual and collective point of view. Many errors occur during these interpretations, which lead to wrong decision-making that can lead to incalculable losses for cities, states and even countries.
One of the main causes of misinterpretations in texts and materials is the erroneously adopted concept of correlation, and how dangerous it can be for the adoption of public policies and decisions with great impact. See, for example, the article in the link: “Possible correlation detected between air pollution and deaths from Covid-19”. Several readers, when analyzing this headline, immediately thought: to combat Covid-19, therefore, we must reduce air pollution, and this will cause a decrease in contagion and, consequently, in the number of deaths from the virus. Thus, these readers, if they held high public positions, would have made wrong decisions, creating policies that would have no effect or satisfactory results.
Why were these readers wrong? Because correlation should never be confused with causation . Saying that air pollution and deaths from Covid-19 are correlated will never be the same as saying that a high level of air pollution causes more deaths from Covid-19. In other words, two variables (in the example, air pollution and deaths from Covid-19) that co-vary do not necessarily have a cause-effect relationship. To make this difference even more evident, see the clueless example from the website tylervigen.com: the very high correlation (remember that the maximum mathematical value of a correction is 1) between the divorce rate in the state of Maine and northern consumption -American per capita of margarine: 0.9926.
Divorces can be considered social and bureaucratic problems, which create more legal processes, thus demanding manpower and costs for public coffers. In this way, public managers must think of ways to reduce divorce rates, and, according to the graph above, the level of margarine purchases must therefore be reduced . Perhaps the biggest result a manager will achieve with this line of reasoning is removal from their position, do you agree?
Weird examples like the one mentioned may be easier to dismiss as wrong, but let's go back to the case of Covid-19: does air pollution cause more deaths from the virus? A public manager who answers “yes” to this question is wrong, and may make decisions that are also wrong. Following this line of reasoning, should the circulation of polluting vehicles (which do) cause an increase in pollution levels be banned, in order to reduce the number of deaths from Covid-19?
Some readers will note an issue, which is the most impressive and ironic of public decisions: this manager may achieve a decrease in coronavirus cases and deaths because of this ban, thus emerging as a hero . But not because pollution levels cause more deaths from Covid-19, but precisely because they are positively correlated, that is, they vary in a similar way. To talk about causality, a third variable must be added to the recipe: social isolation .
It can be said that social isolation causes a decrease in the circulation of people on the streets, which causes a smaller number of vehicles on public roads, which in turn causes a decrease in pollution levels. On the other hand, isolation causes a decrease in interpersonal interaction, which causes a decrease in the rate of Covid-19 contagion, and consequently in the number of deaths. Both headline variables decreasing, thus indicating a correlation, not cause-and-effect .
This is the main tip for analyzing headlines and information that comes to us every day: causality infers that one variable causes the other, and therefore no other is necessary for this relationship to occur. So, when you read news like “Possible correlation detected between air pollution and deaths from Covid-19”, look for other variables that can be associated and, thus, find the real cause-effect relationship. In this case, for example, a high population density causes greater air pollution, and also causes more social interactions and, consequently, greater contagion of the virus . It is not the case that pollution causes more deaths from Covid-19, but rather that the high population density causes both problems. We are bombarded daily with information, some true and some not so much. Critical and analytical thinking must be applied to headlines and news such as the one presented, thus avoiding recurring and extremely important modern problems, such as mistaken decision-making, propagation of false information and support for campaigns that will not have expected effects. When you receive any type of information, especially those that claim causal relationships (medicine x improves situation y, reducing x makes y not happen, among others), always question and look for more realistic answers. In other words, never confuse causality and correlation, or relationship and cause-effect.
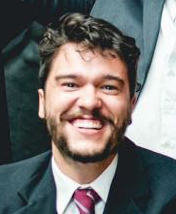